Replyio
The Role of Machine Learning in Advancing AI-Based Reply Generators
-
Aug 10, 2024
-
6 min read
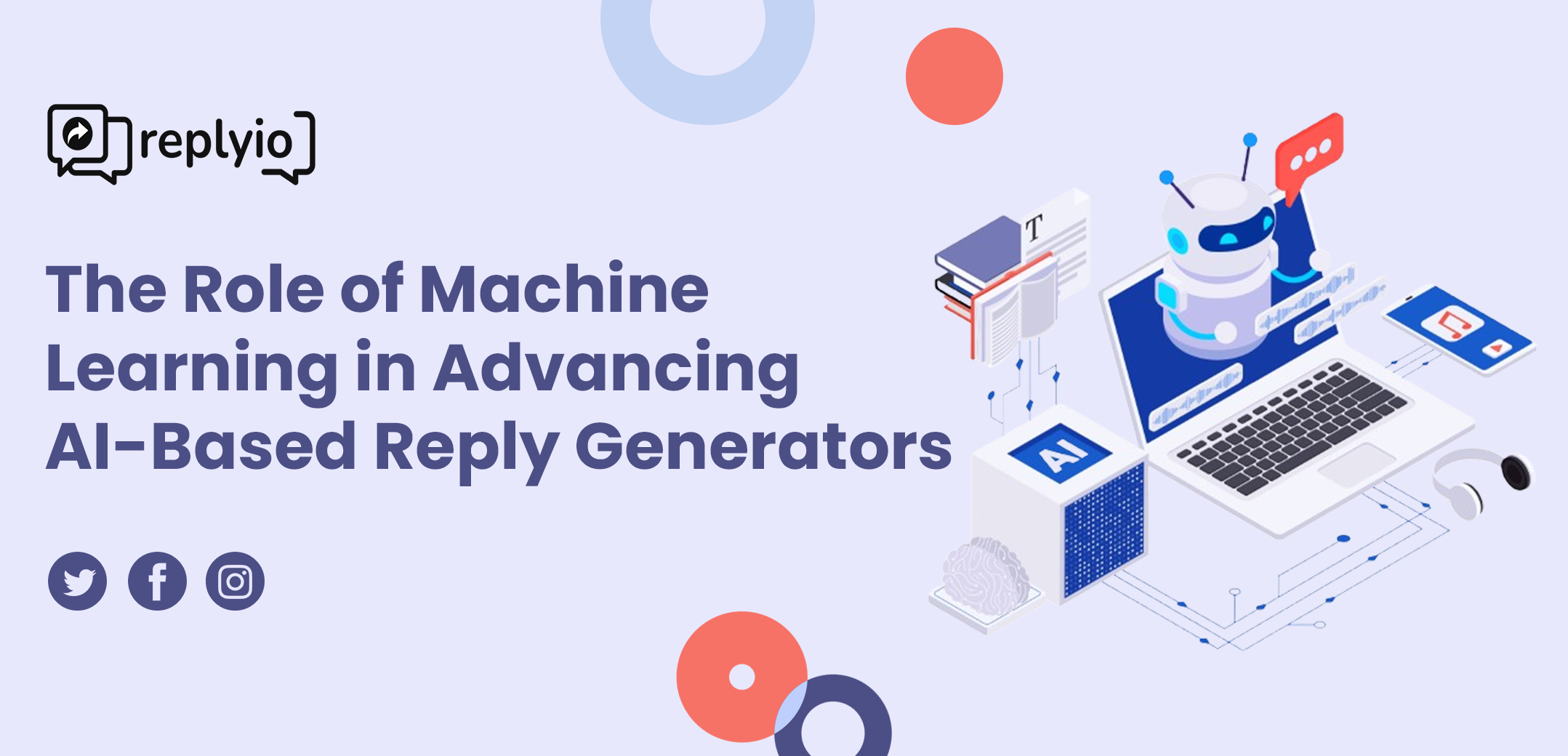
In today’s fast-paced digital world, communication is at the core of everything. Businesses, customer service teams, and marketing professionals all strive to provide timely, personalized responses to their customers, leads, and users. One of the cutting-edge solutions for this is AI-based reply generators, a tool that is growing in both sophistication and adoption. Machine learning (ML) plays a pivotal role in advancing these systems, making them more efficient, accurate, and human-like. But how exactly does machine learning enhance AI-based reply generators? Let’s dive into it.
What are AI-Based Reply Generators?
Before exploring the role of machine learning, it's essential to understand what AI-based reply generators are. These are algorithms that automatically generate text responses in communication channels like emails, social media, and chatbots. Whether it's responding to a customer query or following up on a lead, these systems craft relevant replies based on the input they receive.
The magic lies in their ability to mimic human responses. By analyzing vast amounts of data and understanding context, reply generators can produce personalized, coherent, and contextually relevant answers, freeing up human workers for more complex tasks.
How Machine Learning Elevates AI Reply Generators
1. Context Understanding through Natural Language Processing (NLP)
Machine learning models rely heavily on Natural Language Processing (NLP) to understand the context of a conversation. NLP helps AI systems to parse the syntax and semantics of language, understanding not just the words but the intent behind them. Over time, machine learning algorithms become more sophisticated in recognizing various contexts—whether it's a complaint, question, or feedback.
For instance, when a customer asks, “Can I get a refund?” the AI-powered reply generator recognizes that this is a request rather than a generic inquiry. Machine learning makes this context understanding increasingly accurate as it processes more and more examples.
2. Personalization at Scale
Personalization is the key to meaningful interactions. Machine learning models continuously learn from user behavior, preferences, and past interactions. This allows AI-based reply generators to craft tailored responses rather than generic, one-size-fits-all replies.
Let’s say a customer regularly engages with a specific product feature. The AI reply generator can provide responses that focus on that feature, making the reply more relevant. Over time, machine learning enhances its understanding of individual users, creating more personalized interactions on a large scale.
3. Continuous Improvement through Feedback Loops
One of the most significant advantages of machine learning in AI reply generators is their ability to improve continuously. With every interaction, AI models receive feedback—whether explicit (a user upvoting or downvoting a response) or implicit (how quickly a problem is resolved). These feedback loops enable the machine learning algorithm to fine-tune itself over time, adapting to new patterns and refining its ability to generate effective replies.
Unlike rule-based systems, which rely on static instructions, machine learning allows reply generators to evolve based on real-world data. This leads to more accurate, helpful, and human-like responses.
4. Handling Complex Queries with Deep Learning Models
Basic AI reply generators can handle straightforward inquiries, but what happens when a user presents a more complex question? Here’s where deep learning models come into play. Deep learning techniques, such as transformers (the backbone of advanced language models like GPT), allow AI systems to manage nuanced and multi-faceted conversations.
Machine learning models can break down long, intricate queries into smaller components and generate appropriate replies for each part. This leads to a more intelligent system capable of handling sophisticated scenarios like dispute resolutions or technical support queries.
5. Learning and Adapting to Different Communication Styles
No two people communicate the same way. Some may prefer formal language, while others lean towards casual, emoji-filled replies. Machine learning enables AI-based reply generators to adapt to these differences. By analyzing past interactions, the AI can identify the user's preferred communication style and adjust its tone accordingly. This adaptability is crucial for businesses that interact with a wide range of customers, ensuring the right tone is struck in each interaction.
6. Real-Time Learning and Responsiveness
Another significant advancement that machine learning brings to reply generators is real-time learning. With the help of online learning algorithms, these systems can incorporate new data and adapt in near real-time. For instance, if there’s a sudden surge in inquiries about a particular topic or product, the system quickly learns from these interactions and generates more refined replies specific to that new pattern.
This responsiveness ensures that businesses stay agile and deliver relevant answers as trends or customer concerns evolve.
The Future of AI-Based Reply Generators
Machine learning is the driving force behind the remarkable advancements in AI-based reply generators. From personalized responses to contextually aware interactions and deep learning for handling complex queries, machine learning equips these systems with the ability to function more like human agents.
As the models continue to evolve, AI-based reply generators will become even more accurate, intelligent, and capable of understanding human emotions, thus revolutionizing how businesses and individuals communicate. The future promises even more exciting innovations as machine learning algorithms continue to learn and adapt, bringing us closer to seamless, human-like AI communications.
For businesses, adopting these systems now could mean staying ahead of the curve, enhancing customer satisfaction, and driving more efficient operations. And for consumers, it means better, more personalized experiences with the brands they engage with.